Are “data scientist” and “data engineer” different titles for someone doing the same type of work? Or do they present two different attitudes toward solving the same problems? Since data science has become a buzzword, internet discussions like this one have not stopped. Recently, several bloggers used “Data Engineers versus Data Scientists” as titles for their articles to express their views on these “terminologies” or “professions.”
Some bloggers use an even more complicated title: Data Scientist versus Data Analyst versus Data Engineer. This is to describe differences among all three roles in the data analysis process. Recently, an article by the scientist, Mihail Eric, entitled We Don’t Need Data Scientists, We Need Data Engineers has been widely distributed and discussed on the internet. This article first appeared in January 2021, and according to KDnuggets, the article was the most popular during early February 2021. However, author of another article, posted in BUSINESS INTELLIGIST, disagreed with Mihail’s opinion during that month, and wrote an article titled We Don’t Need Data Scientists?!? to explain his viewpoint. He stated, “I think that the field of Data Science is very far from being mature and therefore we need more data scientists, but we need data scientists who know how to work with data and who have the business acumen to really understand the issues we are trying to solve and to effectively communicate the discoveries back to business audience using the language that the audience can actually understand.” Other people take the middle ground, by stating, “We don’t need everyone to be a data scientist.” The author of this post will refrain from taking sides; however, we should think of our current statistics education and the types of people we train/teach in schools. Would they fit the needs of the community as data scientists, data engineers, or data analysts?
Statisticians are hard-pressed to deal with “big” data. Many schools are eager to have data science departments/programs. To get what they want, they adopt the simplest outlet of replacing the names of their “data science related” departments and/or courses with modern names, but without renovating their content. In doing so, they may attract more students to register for their departments/program in the beginning, but people know that this is like a “new bottle with old wine.” But does the name-changing strategy work?
From the perspective of statisticians, statistical knowledge and methods are essential to data science. However, we know that statistical knowledge and methods alone may not be useful in solving practical problems, due to large data sizes and limitations of application scenarios. We could simply say that these are computational and domain knowledge issues, which are not “directly related” to “Statistics” or “data analysis.” However, this attitude does not assist the scientific/research community and is not useful, as we are members of a problem-solving team. We should dig up the valuable information from data so we can help the world, human beings, the environment, etc. Thus, although Statistics plays a necessary role in Data Science, it is not equivalent to Data Science. More details should be considered, such as computational and domain knowledge. Can we resolve this difficulty and really train all types of students by recruiting computer scientists to join the conventional Statistics/Biostatistics departments?
Recently, many researchers have acknowledged this fact, and some universities have redesigned their entire program, such as University of California, Berkeley, which tried to embed computation and a “data analysis sense” into many traditional courses. Shiga University, Japan, adopted a totally different approach; they designed a new department from scratch; their undergraduate program for Data Science was designed first, followed gradually by the graduate programs. During the first few years of the design of their program, they organized workshops and invited scholars and researchers from other countries to discuss and share their experiences about teaching courses in Data Science.
We now know that changing the titles of departments or courses cannot adequately promote statistics. These universities demonstrate just two examples of taking a more aggressive approach to redesign programs. Besides, for the above mentioned blogger’s article, there are also many discussions on statistics education at conferences. In the IASE 2019 satellite conference, Dr. Richard De Veaux presented a talk, Data Science For All, where he stated that “our intro Stats course is becoming even less relevant to students’ needs,” and “we are teaching the same course that we taught in 1958 …or even 1996.” Most of us know that we should change the conventional statistical program. However, it is problematic to figure out how and what to change.
The bloggers’ articles referred to earlier also remind me of Dr. Hilary Parker’s presentation, one of the keynote speakers of ICOTS 10, 2018, at Kyoto, Japan. The title of her talk was Cultivating Creativity in Data Work where she emphasizes that we should include the ideas of “working with data,” “effectively communicating,” and “domain knowledge” into our Data Science/Statistics programs. She also mentioned that “if Data Science is an art, why don’t we teach it like art?” Thereafter, she used the Peabody Institute Program of Johns Hopkins University to illustrate her thoughts:
- Music theory: Theory
- Instrument: Programming Language
- Composition: Narrative
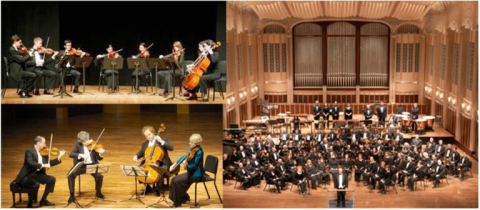
In fact, in most conservatory programs, students must have some ensemble experiences, apart from their own instruments, as members of a two-person ensemble or large orchestra. This type of training allows students to experience leadership and partnership. This is especially so when students play in small ensembles, where leadership and partnership are exchanged frequently, according to the needs of the musical performance.
There are many faces in Data Science, and researchers participate in its research from various perspectives. In the paper of Blei and Smyth — Science and Data Science (PNAS, 2017, Vol 114), Data Science was discussed from the perspectives of scientific research: statistical, computational, and human perspectives. However, from a problem-solving perspective, these are never purely statistical or computational problems; they are usually a mix of varied techniques. Most importantly, solving the problem usually requires excellent domain knowledge, such that the developed or created methods/algorithms are relevant and useful. In Blei and Smyth’s (2017) conclusion, they state “Holistic data science requires that we understand the context of data, appreciate the responsibilities involved in using private and public data, and clearly communicate what a dataset can and cannot tell us about the world.”

To deal with this type of modern data as mentioned in Blei and Smyth’s (2017) requires varied knowledge, including statistical, computing, and most importantly, domain-specific/application-related knowledge. No one person can master these many types of knowledge across different areas. Teamwork is now essential for analyzing the messy and massy data described in modern large data analyses and related studies. However, the goal of a statistics PhD/master’s program, in general, is to train students to work independently to become leaders in their professions. It is rare to have classes that teach students how to work as part of a team, or conduct beneficial collaborations.
Dr. Parker mapped out the core courses of Statistics/Data Science in a program from a conservatory perspective. When analyzing messy and massy data, we encounter many problems at the different data analysis stages: statistical, computational, domain specific, etc. We must know when to play the role of a leader, and when to be a supportive partner; your role within a team will change and it must change according to the progress of the project, so that the project goal can be accomplished. This is similar to the ensemble training of conservatories. Hence, if we extend her idea further by including an “ensemble training” concept to statistics/data science programs, our students can embrace the ability to switch their roles within a team as needed, and will be more welcomed to do so.
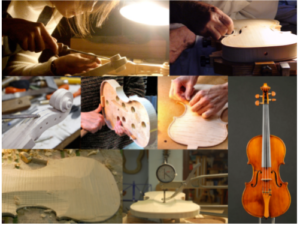
“One man’s technicality is another’s professionalism.” -Kai Lai Chung