ISBIS - Estimation and Group-feature Selection in Sparse Mixture-of-experts with an Application for Exploring Relationships in Human Body Measurements
Date | 02 Jul 2024 |
Time | 14:00 (GMT+02:00) - 15:00 (GMT+02:00) |
Level of instruction | Intermediate |
Instructor |
Abbas Khalili
|
Registration fee | |
Abstract: Mixture-of-experts provide flexible statistical models for a wide range of regression (supervised learning) problems. Often a large number of covariates (features) are available in many modern applications yet only a small subset of them is useful in explaining a response variable of interest. This calls for a feature selection device. In this talk, we present new group-feature selection and estimation methods for sparse mixture-of-experts models when the number of features can be nearly comparable to the sample size. Statistical properties, and numerical implementation of the methods will be discussed. We also demonstrate the performance of the methods through simulations and apply them to a real data to explore relationships in body measurements.
Instructors
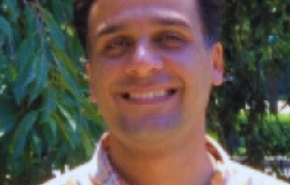
About the instructor
Department of Mathematics and Statistics McGill University - Montreal, Quebec, Canada